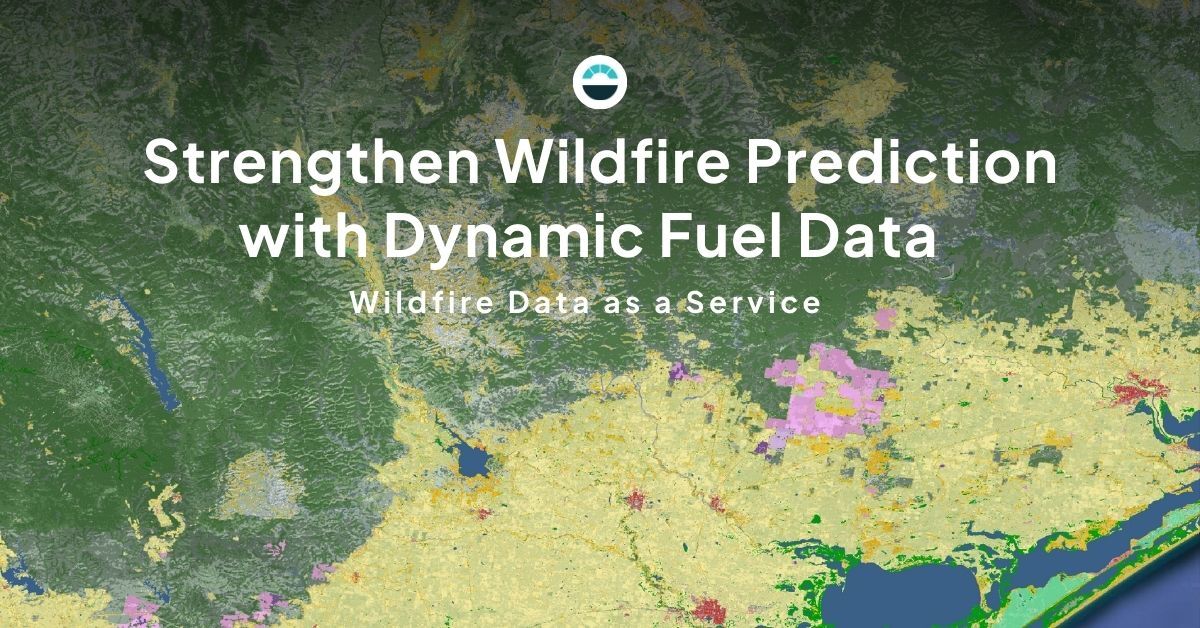
In 2024, there were over 64,800 wildfires in the United States alone, according to the National Interagency Fire Center (NIFC). Globally, wildfires are also on the rise in South America, parts of western Europe, sub-Saharan Africa, and Australia. It’s increasingly crucial for local government, forestry, and fire service leaders to have a granular understanding of fire risks in their area to protect people and infrastructure.
To know where fires could start and how they are likely to spread once they do, fire and forestry leaders need to increase their understanding of fuel in the areas they protect. That’s why our team is pleased to announce the launch of our Wildfire Data as a Service (DaaS) offerings, with a focus on dynamic fuel data for fire behavior modeling.
Moving forward, we’ll…
- Explain wildfire fuel modeling at a high level
- Explore what fuel data is most relevant for wildfire prediction and prevention
- Illustrate how AEM packages wildfire data for use with any wildfire behavior system
What is wildfire fuel modeling?
Fuels are essentially the materials in which fire is carried. Generally, wildfire fuels are living or previously living material whose structure, arrangement and condition make it possible for fire to propagate. Fuel is one of the three sides of the fire behavior triangle, alongside weather and topography.
Wildfire is a phenomenon of context. The more precisely and accurately you can define its context, the higher the chances of successfully predicting its behavior using mathematical formulas created by fire scientists or inferred via AI. Whatever the methodology, fire behavior estimation requires numbers describing the three components of the fire behavior triangle for a given place and time.
So, when we talk about fuel data, we are really talking about our ability to specify the numbers we enter into fire behavior formulas for each fuel type. These numbers are things like fuel load, which is the amount of available fuel to burn based on size and if it’s alive or dead. There are also numbers describing fuel structure and arrangement, like height, surface-to-air volume ratio, and wind reduction factor, among many others.
Wildfire fuel data modeling is the process of combining data from multiple sources to understand the current state of fuel in an area, estimating fuel types and their associated descriptive parameters, and arranging that information in a format that enables robust fire behavior estimation. Dynamic fuel data modeling goes further. It repeats this process more frequently to make sure mapped fuel data descriptions reflect real-world changes in the fuels.
Fuels can change often, sometimes dramatically. Droughts impact fuel loads and arrangement, crops get harvested, grasses grow and die seasonally, wind events or pests can damage forests altering structure and arrangement — and those are just a few of the factors at play.
What data do you need to understand wildfire fuel?
Fire behavior science can be complex, but generally, you need to know:
- Fuel types: What kind(s) of fuel are present in the landscape? Types can be basic like "evergreen needleleaf forest," or where sufficient data exists, they can be more specific, like "dry eucalyptus forest on coastal plains." Satellites are the standard tool for visualizing this, but we still can’t rely on them to create a full picture of fuel characteristics because they can’t see under tree canopies in enough detail. By better defining and more carefully tracking fuel types, we can predict what the satellites can’t see with greater accuracy.
- Fuel structure: Structure is about identifying layers (canopy, elevated layers, surface layers, etc.) and describing what’s in each layer. Structure also contributes to how much wind can penetrate the fuel complex, which can significantly affect fire behavior.
- Fuel arrangement: Arrangement is closely associated with structure and describes horizontal and vertical continuity stand and canopy bulk density. Fuel compressed into a block will burn much differently than the same amount of fuel arranged like cotton candy.
- Fuel condition: How wet or dry is the fuel in the area? The amount of moisture in the fuel and at what moisture threshold fire will extinguish are important descriptors of condition.
…With that said, the elephant in the room is the recency of the data. If you’re using static, representative fuel data that updates infrequently, your fuel data cannot reflect dynamic changes due to the seasons, wind or pest damage, and in particular for forest systems, the impact of drought.
Our approach to wildfire data as a service
AEM’s Wildfire Data as a Service (DaaS) is a subscription service delivering essential landscape characterization data to support wildfire predictive analytics. Our fuel data updates every 10 days to keep wildfire and land managers as up to date as possible on wildfire threats in their area. But it takes more than just fuel data to model wildfire behavior well. Our Wildfire DaaS offering is expanding beyond fuel data, and will include:
- Global fuels: You get a detailed snapshot of wildfire fuels in your area in 20x20 meter resolution, including structural and drought variants with around 200 fuel types available anywhere on the planet. For jurisdictions or regions with appropriate source data who require more detailed base fuel types, we can also offer domain specific fuel data with significantly more detailed types.
- Digital elevation: We can provide digital terrain elevation data (in meters above sea level) to inform the topography side of the fire behavior triangle.
- Fire/disturbance history: Even though the fuels themselves are updated frequently to reflect changes in type, structure and drought, when these fuels burn or are otherwise disturbed, we provide this layer to enable fuel re-accumulation models.
- Wind modifiers: This layer can be used to downscale wind direction and speed data to account for how wind bends and changes speed over terrain.
- Disruptions: Spreading fire encounters obstacles it must breach in order to continue spreading. This layer defines the width of non-burnable breaks and disruptions, often used in fire spread simulators.
- Assets: This layer categorizes manmade buildings and structures used to provide property level house loss probabilities and drive unique fire risk layers.
We know that all data is what you make of it, which is why we package our offerings in a way that makes it easy to bring into any wildfire modeling or simulation software. We’re proud to say our fuel data is:
- Detailed & dynamic: We update our fuel data for all customers every 10 days using a blend of satellite imagery and AI to provide current insights on structural class, pasture biomass, and drought. We also update asset data to account for new buildings and changes in manmade structures monthly.
- System agnostic: Our data structures are extensible and can be delivered with definition files making them compatible with any fire behavior modeling system. We want your team to spend more time focusing on adding value to the tools that work for them, leaving the complex data effort to us.
- Customer controlled: We know that local knowledge is as important to community wildfire resilience as any data source. That’s why we work with you to tailor our data for local use and allow customers to select and refine their own fuel parameters that best represent how fuels exist locally.
- Systematic & consistent: We provide a consistent methodology, data, and format that you can use for modeling, decision-making, training and operating AI, and beyond.
- Bottom-up global coverage: We have the ability to provide our global fuel data subscription for customers anywhere on the planet. Our more specific fuel data will be available for Australia, the USA, Europe, and Canada before year-end 2025.
AEM’s ability to provide accurate, actionable, well-packaged wildfire data anywhere at scale is unmatched. Our solutions are built by wildfire specialists for wildfire specialists in this specific moment. We’re proud to be in a position to support an unprecedented leap forward in wildfire data, modeling, and risk analytics for countries and organizations that have previously been unable to access the most advanced capabilities.
If you'd like to see how better wildfire data could make a difference in your ability to understand, predict, and respond to fires, it's time to sit down for a chat.